Objectives
With this backdrop it was imperative to study the disparities
in length of life by means of Gini Coefficient across
age (0-5, 15-40 and for all ages), sex, over periods
from 1970/71-2005 for India and some of its states based
on geographic location viz. Assam (North-east), Kerala
(South), Maharashtra (West), Orissa (East), Punjab (North)
and Uttar Pradesh (Central). Further these states are
witnessing different stages of development as Maharashtra
and Punjab are considered among the richest states whereas
Assam and Orissa are among the poorest states. Though
Punjab has the lowest infant mortality rate after Kerala
the gender gap is more pronounced in Punjab. Uttar Pradesh
is considered as the most populous state and Kerala
holds its position as the educationally and socially
developed state. Thus it will suffice one to observe
the performance of better and worst states in the health
inequality scenario. The aforesaid components of age
will help in studying the overall variation in degrees
of inequality in length of life at different ages. The
justification of using the periods from 1970/71-75 to
2001-05 is the non availability of reliable data prior
to these periods, since Sample Registration System is
the only unique source of information providing representative
and the most complete data on mortality in India. (National
Population Commission, 2001). This work further studies
the relation between inequality in the length of life
and average length of life for all states and age groups.
Data and methodology
The data used for computing Gini coefficient was secondary
provided by Sample Registration System (SRS). The office
of the Registrar General of India started the process
of sample registration of births and deaths in India
in 1964-65 on a pilot basis and on a full scale from
1969-70. This scheme was based on dual record system
in India. It provided unswerving estimates of birth
rate, death rate and infant mortality rate for rural,
urban and combined areas for India and for some of its
states and union territories. An abridged life table
had been constructed by the office of Registrar General
of India for males and females from 1970 -75 to 2001-05
for India and some of its major states using SRS data.
However life tables were constructed for 1971-75 instead
of 1970-75 for the states Punjab and Kerala. In this
study we utilized these life tables to calculate Gini
coefficient.
The Gini coefficient was developed by the Italian statistician
Corrado Gini and published in his 1912 paper "Variabilità
e mutabilità" ("Variability and Mutability").
It is based on the Lorenz curve, a cumulative frequency
curve that compares the empirical distribution of a
variable with its uniform distribution represented by
a diagonal line. The greater the area included between
the Lorenz curve and the diagonal, the greater the inequality.
A small value of Go indicates equality in income distribution
and larger value specifies inequalities. In case of
inter individual differences in length of life, it is
equal to zero if all individuals die at the same age;
and equal to one if all people die at age zero and one
individual dies at an infinitely old age. (Shkolnikov,
et.al ,2001). Though Gini coefficient reflects the level
of inequality it is generally used for comparative purposes.
Gini index has many different formulations and interesting
interpretations. All of them are equivalent. (Anand,
1983). Hanada (1983) defined Gini coefficient as:
Whereas
l(x) is the number of survivors at the beginning of
the interval x.
l(0) is the size of the birth cohort.
e(0) is the life expectancy at birth.
This formula was used to compute Go
for full range of ages. However for limited range of
ages this formula can be rewritten as
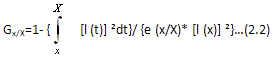
Where the temporary life expectancy is
(Namboodiri and Suchindran, 1987).
is the number of person years lived by the l(x ) persons
during the interval (x, x+n).
T(x) is the total number of person years lived during
the interval (x, x+n).

denotes the number of deaths during (x,x+n).
is the fraction of years lived by those dying in the
interval (x,x+n) on the average.
Chiang (1960a) observe that nax is more or less invariant
with respect to sex, race, cause of death, geographic
location, and other demographic variables. He further
remarked that 
computed for a population for each age group can be
used for many populations. For calculating
in our study a set of Chiang's estimates of nax (Namboodiri
and Suchindran, 1987, pg 26) was used. For the extremely
old ages
is taken as 0.5.
The integral part of equation
2.2 is given by

is the mortality rate.
And
Since for the first year of life l(x) falls more steeply,
so the equation (2.4) will not be suitable for finding
(Shkolnikov, op.cit, 320). Thus J. Borgois-Pichat (1951)
formula
was used.
The last
age group used in the mortality data as given by SRS
was 70+. Hence we apply mortality
law 
to extrapolate survivors in a life table beyond the
final age as discussed by Preston et.al (2001). Parameters
C, a and b can be estimated from the last three values
of the life table survival function, l(y), l(y+n) and
l(y+2n).(Horiuchi and Coale,1982).
was defined to find a solution for open ended interval.
Finally Go was computed by using
equation (2.2).
Results and Conclusions
From column (2) of Table 1 one
it is observed that inequality in the length of life
among male population of India decreased from 0.3019
in 1970-71 to 0.1673 in 2001-05. On the other hand its
female population decrement was from 0.3323 to 0.1826
as portrayed in Table 2. However from the two tables
one observed that Gini coefficient was higher among
the female population as compared to the male population.
The negative correlation coefficient between life expectancy
at birth and Gini coefficient for India across periods
was -0.74 for men and -1 for women as shown in Table
7. The correlation coefficient -0.74 among men indicated
a firm negative linear relationship between the variables.
The value -1 for females represented the perfect negative
linear relationship as the two variables moved in opposite
direction with the same amount. The value of correlation
will depend on the mortality levels of the countries
and periods. A higher value of correlation coefficient
will reflect the divergence in the levels of mortality.
(Shkolnikov, et.al, 2003). Column (3) of the same tables
gave the computed Gini coefficient values for the male
and female populace of Assam. A higher Go value was
observed for Assam as compared to Go value of India
for both male and female populace. The negative associations
between the two indicators for the aforementioned periods
for the male and female inhabitants were -1 and -1 respectively.
The third state considered here was Kerala, where Go
was represented by (4) column of the aforesaid tables.
A smaller value of Gini coefficient was observed for
both male and female population of Kerala as compared
to other states including India. The variability in
the length of life declined from 0.1860 in 1971-75 to
0.0918 in 2001-05 among males of Kerala. It could be
deduced from column (4) that Go value for female populace
for all ages reduced from 0.1742 in 1971-75 to 0.0478
in 2001-05. Thus among Kerala populace one observed
that inequality in the age of death was small among
females as compared to the male people. The correlation
coefficients swung from -0.99 to -1 between male to
female inhabitants of Kerala across the periods. Maharashtra,
being a wealthy and developed state its Gini coefficient
value ranged from 0.2687 to 0.1419 among male members
and its female counterparts ranged from 0.2697 to 0.1216
across the aforesaid periods. Thus the inequality was
higher as compared to its fellow state Kerala. The correlation
was -0.99 both for males and females of Maharashtra.
The next state Orissa, experienced highest Gini value
as compared to all the states and India among its male
members. In 1970-75 Gini coefficient for male populace
was 0.3407 and it reduced to 0.2135 in 2001-05. A higher
Gini value of 0.3525 was observed for women of Orissa
in 1970-75 and lessened to 0.2187 in 2001-05. The correlation
value ranged from -0.92 to -1 among males and females
of Orissa. Gini coefficient for the male and female
population of Punjab for the period 1971-75 were 0.2257
and 0.2509 which reduced to 0.1392 and 0.1307 in 2001-05
respectively. Though Punjab was an economically developed
state of India a contrast higher value of Gini coefficient
was visualized among the female population as compared
to its male populace except for the periods 1986-90,
1991-95 and 1996-2000. This explained the gender inequality
in the age at death. The negative association between
life expectancy at birth and Gini coefficient for all
ages across the periods were -0.99 and -1 among men
and women of Punjab. From column (8) we inferred that
Go value decreased from 0.3000 in 1970-75 to 0.2018
in 2001-05 among male inhabitants of Uttar Pradesh.
However for female population the same column depicted
that these values decreased from 0.3484 in 1970-75 to
0.2220 in 2001-05 for all ages. Thus one deduced that
inequality in the age of death was more among female
inhabitants of Uttar Pradesh in comparison to males
of Uttar Pradesh The last entry of columns ((8) were
0.2018 and 0.2220 respectively for the period 2001-05
of male and female population of Uttar Pradesh, which
was larger as compared to 0.1860 and 0.1742 for both
male and female population of Kerala for the period
1971-75. Though Go value decreased
across periods for male and female population of Uttar
Pradesh a larger Go was observed
for this populace as compared to other states including
India and this leads to larger inequality in the length
of life. The correlation coefficient between eo and
Go among the male and female population of Uttar Pradesh
were -0.92 and -0.91 respectively.
The inequality among individuals depends on many factors.
One such factor was age, since some age groups would
have more tendency of dying as compared to other age
groups. Choudhury et.al. (2007) viewed that probability
of an individual afflicted by various chronic diseases
differed across ages. Ho et.al (2009) studied the characterization
of international variation in mortality rates by age
in 2005 in the United States and observed that death
rates rank poorly between ages 40-75 among males and
females of US. Further Caselli (2010) proved that mortality
pattern by cause diverges as one moved from infant to
adult age and from adult to old age. This incites one
to obtain inequality in the length of life among age
groups 0-5 and 15-40 for all states by computing Go.
Tables 3 and 4 measured the Gini coefficient across
different periods, among different states, for both
male and female populace irrespective of region for
age group 0-5. A similar pattern of diminishing inequality
in the length of life was observed across the periods.
However all Go values of Tables
3 and 4 were smaller than Gini coefficient of Tables
1 and 2. The same trend of larger Go
value for Orissa and Uttar Pradesh and smaller for Kerala,
was seen. The same mode of smaller Go was perceived
among males as compared to females for all states except
Kerala. Further, the association regarding Gini coefficient
and average length of life can be elucidated similarly
as discussed earlier.
Tables 5 and 6 portrayed G15 values
for age group 15-40 for population across all periods
and for different states as discussed earlier. The G15
values for the male population of India decreased from
0.0349 in 1970-75 to 0.0183 in 2001-05. And for its
females it was from 0.0502 in 1970-75 to 0.0261 in 2001-05.
The correlation coefficient between inequality in the
length of life and expectation of life at age 15 among
male and female inhabitants of India were -0.94 and
-0.99. Columns 3 of Tables 5 and 6 presented the G15
value for the male and female people of Assam. Gini
coefficients for the male and female inhabitants of
this place for the period 1971-75 were 0.0387 and 0.0727
reduced to 0.0324 and 0.0391 in 2001-05 respectively.
The correlation between the two measurements of life
is -0.86 and -0.97 among men and women of this place.
A similar pattern of diminishing inequality was observed
from column 4 across the periods among the populace
of Kerala. The female inequality in the length of life
diminished from 0.0211 to 0.0097, while for males
it decreased from 0.0232 to 0.0153 across the periods.
The correlation coefficients varied from -0.90 to -0.93
between male to female inhabitants of Kerala across
the periods. The arguments regarding smaller G15
value among the female members of Kerala as compared
to its males would be same. The state Maharashtra experienced
Gini value 0.0319 in 1970-75 whereas it reduced to 0.0242
in 2001-05 among the male members. A slightly higher
Gini value was observed among the female members as
depicted in Table 6. In this age group the state Orissa
has larger Gini coefficient among all the states both
for male and female population. The correlation coefficients
are -0.72 and -0.09 among the male inhabitants of Maharashtra
and Orissa respectively. The female counterparts had
-0.26 and -0.98 correlation coefficients of the aforesaid
states. The next state is Punjab, represented by the
7th column of the same table. The Gini coefficient value
for the period 2001-05 among men and women of Punjab
were 0.0266 and 0.0168 while G15
of 0.0283 and 0.0297 were observed for the period 1971-75.
The same table indicated that Gini coefficient for all
the periods except 1970-75 among males of Punjab was
larger as compared to its female counterparts. Thus
this showed the favourable change among the female populace
of Punjab as less inequality in the length of life was
observed. The correlation coefficients were 0.02 and
-0.92 among male and female populace. A contrast positive
correlation coefficient of 0.02 among males of Punjab
might be due to the amount of change in G15
and e15 across the periods, which
was not uniform. The correlation coefficient 0.02 indicated
a very weak positive unstable linear relationship. Also
the correlation would be weaker if life expectancy continued
to increase while the decline in the inequality in the
length of life had slowed down or stopped. Thus the
correlation coefficient will be weaker if the comparable
levels of mortality are selected. (Shkolnikov, et.al,
2003). The Gini coefficient values for the male and
female inhabitants of Uttar Pradesh were 0.0289 and
0.0363 in 2001-05, which was larger as compared to 0.0232
and 0.0211 in 1971-75 of Kerala. Thus inequality in
the length of life was more significant among the members
of Uttar Pradesh. The association between expectation
of life at age 15 and Gini coefficient among men and
women of Uttar Pradesh across the periods were -0.91
and -0.86. The explanation regarding the value of this
association would be same as above.
From the tables one might conclude that inequality in
the length of life was more apparent among children
of age group 0-5 as compared to its adult populace.
Further a larger Gini value was observed for the female
population of India and its states except for Kerala
as compared to males. In all the tables we viewed that
inequality is least in Kerala and Assam got a higher
rank in disparity in length of life followed by Orissa,
Uttar Pradesh, Maharashtra and Punjab in 2001-05. Similarly
in other periods 1970/71-75 to 1996-2000 the states
Uttar Pradesh, Assam and Orissa are considered as the
most health inequality states according to their Gini
figures. The inhabitants of India and its states also
experience the same kind of variation in age specific
mortality rate as observed in case of inequality in
the length of life. Hence the notion presented by Shkolnikov,
et.al. (2003) that inequality in the length of life
is influenced by the difference in the age specific
mortality rate is substantiated by the present study
too. In India these variations in the age specific mortality
rate is due to the epidemiological transition from the
communicable diseases to AIDS. The infectious, chronic
diseases and rise in injuries/ accidents accelerates
these disparities among the people. The HIV prevalence
rate is more prevalent among the male adults of age
group 15-49. (National Family Health Survey 3, 2005-06).
Further data from SRS depict that Crude Death Rate (CDR)
of India has been declining from 14.9 in 1971 to 7.6
in 2005 in the last three decades. It has also been
observed that the crude death rate is higher than the
national average in Orissa (9.2), Assam (8.6) and Uttar
Pradesh (8.5) whereas Kerala (6.8), Maharashtra (6.6)
and Punjab (7) have lower CDR as compared to India.
(SRS, 2007). An identical pattern has also been observed
in case of maternal mortality rate. A higher value of
maternal mortality rate is seen for the states Assam
(390), Uttar Pradesh (359), and Orissa (258) i.e. above
the national average of 212. (SRS, 2011). However the
states Kerala (81), Maharashtra (104) and Punjab (172)
have done better in maternal mortality indicators than
India. A similar trend is noticed for neonatal, infant
and under 5 mortality rates. ((National Family Health
Survey 3, 2005-06). Bhat (1987) also observed that pace
of mortality reduction is not uniform among the states
of India. Hence in our study we observed a smaller value
of Gini for the states whose infant mortality rate is
lower. Thus the view of Shkolnikov, et.al. (2003) that
reduction of infant mortality rates caused equalization
of age at death is also observed in this study.
The foregoing discussion leads this study to the conclusion
that in India, disparity in health was found according
to geographical region, gender, age groups and among
the periods. This was catalysed by the variation in
assessing health services due to socio-economic, gender
and geographical distance in a populous country like
India. Thus health inequalities were marked from the
start of life and continue into death. (Graham, 2004).
These unfair and avoidable differences in health status
could be bridged by accompanying growth with more equitable
access to the benefits of development to cease the unacceptable
threat to human well being and security. This kind of
analysis might help in identifying the pace of improvement
among the states, across time periods and to check the
benefitting index of population from the existing social
arrangement.
Tables
Different tables have been provided in this section.
Table 1: Go
for Male Population of All Ages
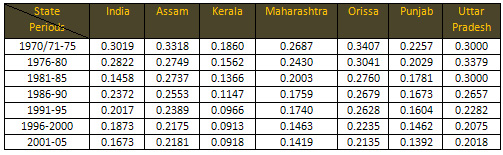
Table 2: Go
for Female Population of All Ages
Table 3: Go
or Male Population Aged 0-5
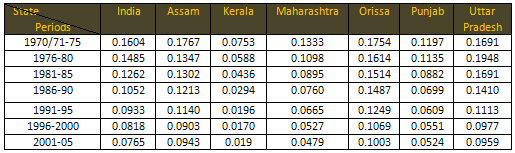
Table 4: Go for Female Population
Aged 0-5
Table 5: G15
for Male Population Aged 15-40
Table 6: G15
for Female Population Aged 15-40
Table 7: Correlation Coefficient between Gini Coefficient
and Expectation of Life
References
Anand, S. (1983): Inequality and Poverty in Malaysia:
Measurement and Decomposition, Oxford University Press,
New York.
Arriaga, E. (1984): Measuring and explaining the change
in life expectancies, Demography 21(1):83-96.
Bhat, M.P. (1987): Mortality in India: levels, trends,
and patterns, A dissertation in demography, Ann Arbor,
Michigan: UMI.
Bourgeois-Pichat, J. (1951): La mesure de la mortalite
infantile. Principes et methodes. Population, 2: 233-248.
Caselli, G., Marsili, M. (2010): Increasing longevity
and decreasing gender mortality differentials: new perspectives
from a study on Italian cohorts, European Population
Conference, Vienna, Austria.
Choudhury, L., Deka, M. and Choudhury, A. (2007): A
Study on Prevalence of Chronic Diseases in Guwahati
City', Assam Statistical Review, vol-21, No. 2, September,
144-169.
Das Gupta, M. (1987): Selective discrimination against
female children in rural Punjab, India, Population and
Development Review 13(1), 77-100.
Dreze, Jean and Gazdar, H. (1996): Uttar Pradesh: The
Burden of inertia, in Dreze and Sen.
Evans, T. Peters, F. (2001): Ethical dimensions of health
equity, In: Evans T et al., eds. Challenging inequities
in health: from ethics to action', New York, Oxford
University Press.
Gakidou E.E. King, G. (2002): Measuring total health
inequality: Adding individual variation to group-level
differences, International Journal for Equity in Health,
1(3).
Goldberg, J., Hayes, W., and Huntley, J. (2004): Understanding
Health Disparities, Health Policy Institute of Ohio.
Government of India. (2002): Tenth Five Year Plan, 2002-2007
Volume II, Sectoral Policies and Programmes, Planning
Commission, New Delhi.
Graham, H. (2004): Socioeconomic Inequalities In Health
In The UK: Evidence On Patterns And Determinants, A
Short Report For The Disability Rights Commission, Institute
for Health Research Lancaster University.
Hanada, K. (1983): A formula of Gini's concentration
ratio and its application to life tables, Journal of
Japan Statistical Society, 13:95-98.
Ho, Jessica Y. and Samuel H. Preston. (2009): US Mortality
in an International Context: Age Variations, PSC Working
Paper Series PSC 09-04.
Horiuchi, Sh., Coale, A.J. (1982): A simple equation
for estimating the expectation of life at old ages,
Population Studies, Vol. 36(2), pp. 317-326.
International Institute for Population Sciences (IIPS).
(2007): National Family Health Survey 2005-2006, India.
Mumbai, India: International Institute for Population
Sciences (IIPS) /ORC Macro International.
Lauridsen, J. and Pradhan, J. (2011): Socio-economic
inequality of immunization coverage in India, Health
Economics Review, 1:11.
Maternal & Child Mortality and Total Fertility Rates.
(2011): Sample Registration System (SRS); (2011) Office
of Registrar General, India.
Namboodiri, K., Suchindran, C.M. (1987): Life table
techniques and their applications, Academic Press, INC,
Harcourt Brace Jovanovich, Publishers.
National Human Development Report. (2001): planningcommission.nic.in/reports/
genrep/ nhdrep/nhdch2.pdf
National Commission on Population. (2001): Report of
the Working Group on registration of births, deaths,
and marriages, New Delhi: National Commission on Population.
Office of the Registrar General and Census Commissioner.
(2001): Census of India, 2001. New Delhi: Office of
the Registrar General.
Office of the Registrar General and Census Commissioner.
(2011): Provisional census Results, 2011. New Delhi:
Office of the Registrar General.
Pal, P., Ghosh, J. (2007): Inequality in India: A survey
of recent trends, Economic and Social Affairs DESA Working
Paper No. 45: ST/ESA/2007/DWP/45.
Saikia, N., Jasilionis, D., Ram, F., Shkolnikov, V.
M. (2011): Trends and geographic differentials in mortality
under age 60 in India, Population Studies 65:1, 73-89.
Sermet, C. Cambois, E. Caselli, G., Vallin, J. Wunch,
G. (2006): Measuring the state of health: Demography:
Analysis and Synthesis. A treatise in population, Academic
Press.
SRS based Abridged Life Tables. (1970-75): Vital Statistics
Division, Office of the Registrar General, India Ministry
of Home Affairs, New Delhi.
SRS based Abridged Life Tables. (1976-80): Vital Statistics
Division, Office of the Registrar General, India Ministry
of Home Affairs, New Delhi.
SRS based Abridged Life Tables. (1981-85): Vital Statistics
Division, Office of the Registrar General, India Ministry
of Home Affairs, New Delhi.
SRS based Abridged Life Tables. (1986-90): Vital Statistics
Division, Office of the Registrar General, India Ministry
of Home Affairs, New Delhi.
SRS based Abridged Life Tables. (1991-95): Registrar
General, India New Delhi.
SRS based Abridged Life Tables. (1996-2000 and 1997-2001):
Registrar General, India New Delhi.
SRS based Abridged Life Tables. (2001-05): Office of
the Registrar General, India New Delhi.
SRS, Bulletin Sample Registration System, Registrar
General, India. (2006): Volume 40 No.1
Shkolnikov, V. Valkonen, T., A. and Andreev, E. (2001):
Measuring inter group inequalities in length of life,
Genus, LVII(3-4):33-62.
The world health report. (2000): Geneva: WHO; 2000.World
Bank. (1996): Improving Women's Health in India. Washington,
D.C
|